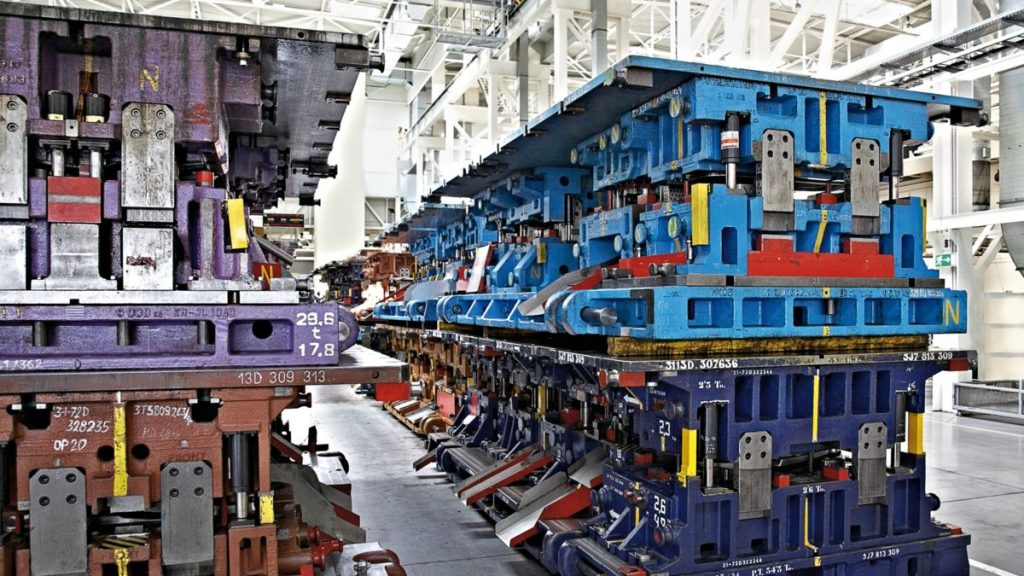
Efficient and resource-saving production
Using anomaly detection in press shops allows early problem detection and proactive maintenance, minimizing downtimes and ensuring product quality, as demonstrated by Changan Automobile.
Automotive companies face global competitive pressure; therefore, they are driven to seek efficient production methods. Moreover, as innovation leaders, they face social and political expectations to build Net Zero factories. Car production, for instance, starts with pressing metal plates into molds to form body parts, a crucial and energy-intensive step. Consequently, this process impacts product quality and can cause significant production delays and cost increases if issues arise.
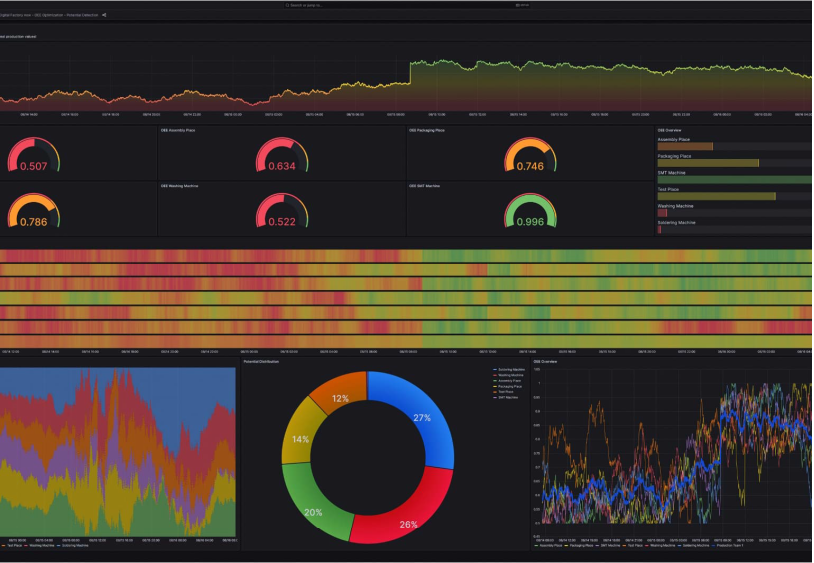
Recognizing the importance of the pressing process, Changan Automobile sought anomaly detection for its pressing plant motors. Consequently, Phoenix Contact’s Digital Factory provides comprehensive digitalization solutions, including MLnext, which simplifies the use of machine learning. As a result, with anomaly detection, Changan can now identify motor issues early, thereby minimizing downtime and ensuring product quality.
Central recording of all engine data and storage in a database
The first step in any machine learning project is creating a database. At Changan, data from two production lines with four presses each was recorded. Phoenix Contact’s IIoT framework, a scalable interface between OT and IT, collects data from various sensors using protocols like Profinet, MQTT, and Modbus. This data is stored in databases like MySQL or InfluxDB, or in the cloud via Proficloud.io, AWS, or Azure, ensuring it’s always available in a comparable form.
Additionally, Changan uses Phoenix Contact’s EMPro device to record motor electrical parameters, including power consumption and performance, via Modbus/TCP. A combination sensor measures temperature and vibration, transmitting values via Profinet IO-Link. The IIoT framework on an EPC 1522 edge PC collects and archives data centrally, with the first database ready in one month.
Simple training of an ML model without programming knowledge
An initial ML model was generated using this database. MLnext Creation’s intuitive interface allows training without programming or statistical knowledge, guiding users step by step. In the Changan project, data was imported, and the “anomaly detection” application was selected. The complexity of the hyperparameter search, determining the number of models trained and compared, was chosen based on available computing time and power. All further steps, like data pre-processing, occur in the background.
The MLnext Creation model is an autoencoder neural network that compresses input data to key features and reconstructs it. For anomaly detection, it’s trained with normal motor data. If an anomaly occurs, the autoencoder can’t fully reconstruct it, leading to significant deviations that identify anomalies.
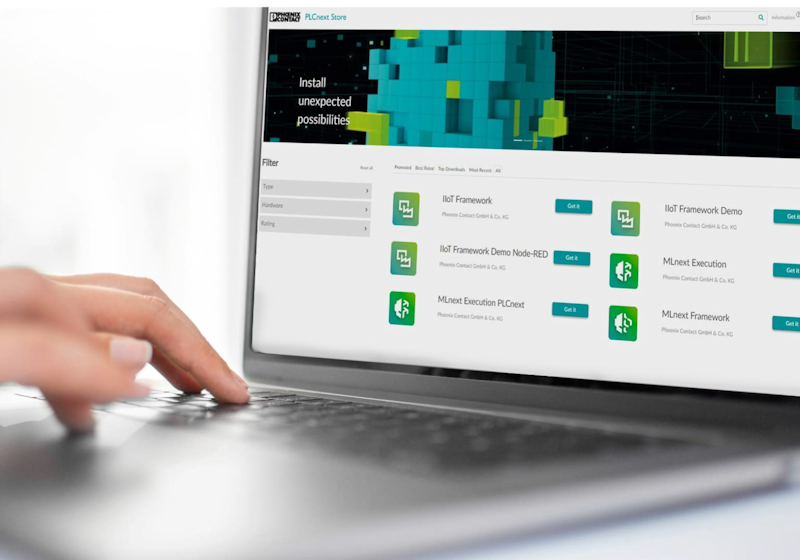
Target group-specific creation of customizable dashboards
They then use the trained model in production. Specifically, MLnext Execution provides a configurable pipeline for data acquisition, pre-processing, model inference, and event storage, all without programming knowledge. In the Changan project, it runs on the same EPS 1522 Edge PC as the IIoT framework, thereby ensuring seamless integration. Consequently, the system loads motor data from the database in near real-time, pre-processes it, analyzes it for anomalies, and archives the results back in the database.
Grafana visualizes the model’s data and results, creating target-specific dashboards for motor data and detected anomalies. It allows Changan to independently modify dashboards and set alarms to alert maintenance personnel to potential issues.
Continuous improvement of the model
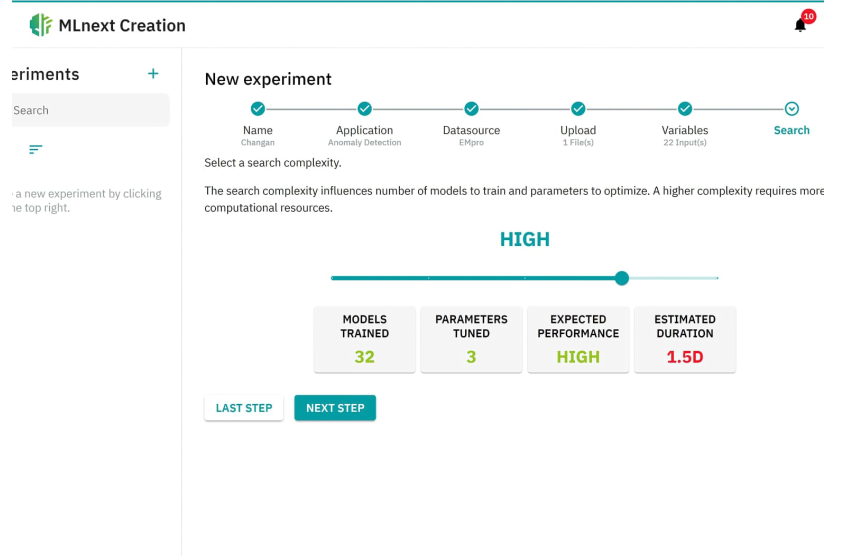
MLnext detects anomalies in Changan Automobile’s press shops early, minimizing downtime and boosting competitiveness. The iterative machine learning process continuously improves the model by evaluating detected anomalies, enhancing detection precision.
Transformation of existing factories into fully digitized production facilities
The automotive industry faces pressure from complex manufacturing and high legal requirements. Phoenix Contact’s Digital Factory now portfolio offers products, solutions, and services to transform factories into fully digitalized, sustainable production facilities. Key advantages include:
- Holistic approach: Collecting, storing, and analyzing data
- Increased efficiency: Reduced downtimes and maintenance workload through data analysis
- No ML expertise required: MLnext simplifies machine learning use
Adaptability: Flexible solutions for various production environments
Read more about Anomaly detection in the press shop of Changan Automobile.